Subscribe to our monthly newsletter to get the latest updates in your inbox
The world is swimming in data. With the increasing prevalence and availability of resources that can facilitate collecting and analyzing massive amounts of data, it's not surprising that more and more businesses are investing in resources that can help make sense of the intimidating data landscape.
However, just as I'd rather swim in the crystal clear waters of the Maldives than in a nasty sewage pond, there are varying levels of data quality. These differences can affect businesses' ability to extract valuable insights from their data and can mean the difference between a worthwhile investment with a competitive advantage and a colossal waste of resources causing setbacks.
Luckily, resources are available to collect, analyze and use good, quality data and your Adswerve friends are ready and eager to help you! This article will discuss data maturity and tools that can help you start on the path to data maturity.
What is data maturity?
I’ve spent some time trying to come up with a good definition for data maturity. My efforts to do so yielded this definition:
Data Maturity is the quantity and quality of data with relation to the ability to use it. In other words:
Data Quality + Data Quantity = Capability
What does this mean? Let's break it down.
Data Quantity
Data quantity represents the amount of data. A low quantity of data means we don't have very much and a high quantity of data means we have a lot of it. Why is quantity important? Great question! Imagine teaching a child about the differences between dogs and cats when they have never seen either in person. You show them a picture of several dogs.
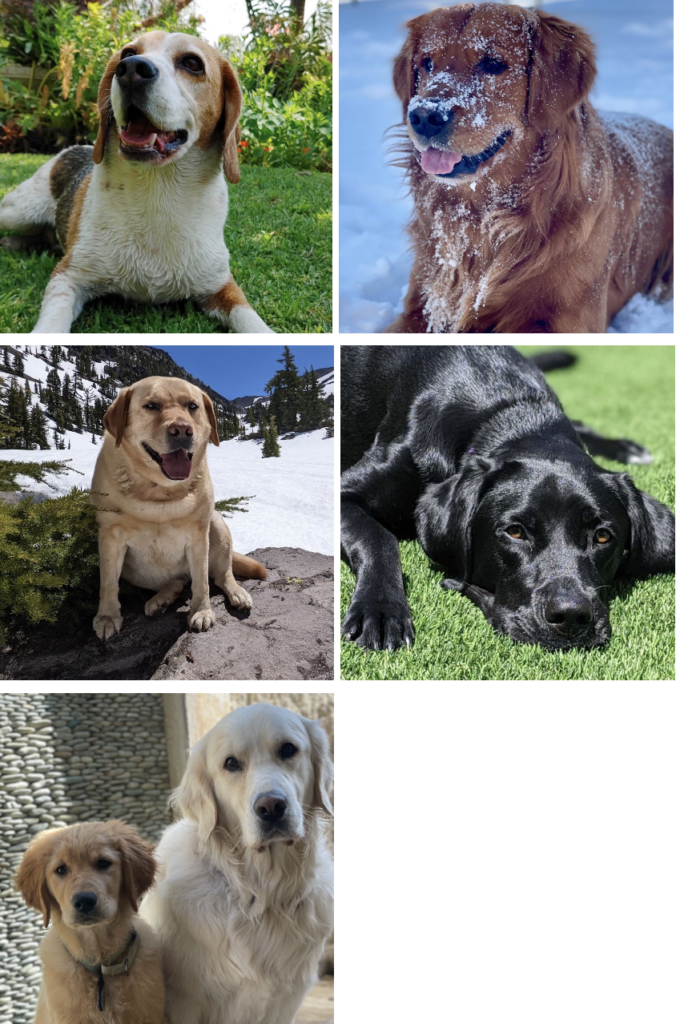
Then you tell the child, "Here is your quiz!" Showing them a picture of this cat, you ask, "Is this a dog or a cat?".
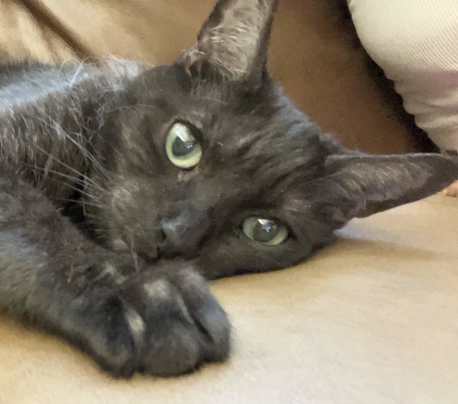
What will the child say? Probably "dog" because they’ve only seen pictures of dogs and have no idea what a cat is! After they predict wrong, you correct them and explain that this is, in fact, a cat. They lock that information away for future predictions. Then, you show them a picture of this dog.
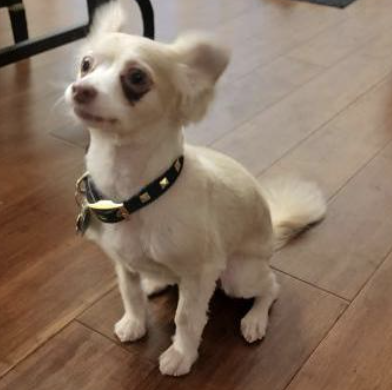
They might say it's a cat. After all, it has pointy ears and it seems to be smaller than the other dogs they’ve seen. No matter what they guess, they’ll be unsure because it looks quite different from the other breeds of dogs and the one cat that they’ve seen before.
This analogy translates to any other type of data efforts. If we’ve only collected a little bit of data, we’re very limited in what we can learn from that data. Humans have a natural ability to generalize pretty quickly, but computers need a lot of data to accurately ascertain insights about a given dataset.
It's important that we not only have a large amount of data, but the distribution of our data reflects reality. As we saw with our initial dog dataset, they all looked pretty similar and we were missing cats altogether. Even our dog dataset, though, made the prediction for the small dog in the child's quiz difficult, as its attributes varied quite a bit from the other dogs. We must make sure that our dataset is large enough to facilitate generalization.
Similarly, it would be very hard to build something like a mixed media model on one month of data. In the marketing sector, one month is simply not enough time to understand the habits and patterns of users. However, having one to two years of data should give us a pretty good idea of how users behave. It should also give us data on unique cases so that the systems we build with the data can generalize well.
Data Quality
Data quality refers to how "good" the data we’ve collected is. Quality has a lot more that goes into it than quantity does. Data quality considers the type of data we’re collecting. Is it pertinent to our use case? I wouldn't want to collect data about dogs' ability to smell when I’m actually interested in understanding how high cats can jump. Similarly, we don't want to collect data that has nothing to do with our business case.
Furthermore, data quality can take into account the accuracy of data. Is the data we’re collecting reflecting the truth? This can be thought of in two ways: is this data reflecting what actually happened, and is this data being influenced or using bias in its measurement methods?
For example, perhaps we told the child that the picture of the small dog was in fact a cat. That would be inaccurate data that the child would use to make incorrect future predictions. Furthermore, our dataset shows bias. We are biased toward a certain look of dogs. Notice the long snout with floppy ears and droopy eyes of each of the dogs in our original dataset. There is not enough representation for dogs with pointy ears or brighter eyes. This would affect our perception of a dog in the future. After all, small dogs and dogs with pointy ears are still dogs!
High-quality data will be accurate and represent reality in a way that doesn't push us to believe one thing or another. I shouldn't assume that all dogs look similar to the dataset I've seen because that’s not the truth. Similarly, I shouldn't assume that all cats have black fur and green eyes. Our data efforts will yield results consistent with the quality of the data. If you want quality results, ensure you have quality data.
Capability
Once we have enough data, and that data is of high quality, we can use it in valuable ways like:
- Data Analysis
- Understanding how users interact with your marketing efforts
- Understanding how users with different demographics feel about your company
- Viewing common paths that users take through your website
- Understanding how loyalty programs affect the user experience
- Determining conversion rates
- Machine Learning
- Modeling user behavior
- Making predictions on users who will convert soon
- Making predictions on users who will churn soon
- Understanding which marketing mediums seem to yield the best results
- Modeling the effectiveness of different website features
- Determining the most effective way to distribute ad spend across channels
- Data Visualization
- Creating dashboards that allow users to interact with the results of any of the above
- Visualizations that help non-technical stakeholders understand the massive amounts of data
Now What?
We've discussed the value of good data. However, how do we approach this seemingly colossal responsibility? How can we start collecting data that is of high quality and how do we get a lot of it? Adswerve's expert team addresses this exact question frequently. While the roadmap for data collection might look a little different for every individual business, here’s an idea for getting started:
- Make a list of all of your data sources. This includes your website, any databases of historical sales, your historical marketing campaigns and their results, spreadsheets you've used to track data about your business and anything else you can think of.
- For each of those data sources, start identifying if the information you can get from those sources would be valuable for your future marketing goals. Understanding how people interact with your website would probably be incredibly valuable, while information about a billboard advertisement that you ran in the early 90s might not be so valuable.
- Once you‘ve identified the valuable data sources that you have access to, investigate how you can collect high-quality data from them. Hint: the Google Marketing Platform (GMP) has a lot of products that can facilitate this data collection with minimal effort;- just "turn them on" with the click of a few buttons.
- For data you can’t collect through GMP products, try building out a first-party data collection system. Are there things your customers are willing to share with you that you might not get by default from GMP products? Build out a database of that information so you can target your audience more specifically.
- Once you have data collection processes in place, be patient. Monitor the data coming in for quality assurance and address issues that may come up.
Each business has its own needs and will have unique situations. Adswerve is here to help! Please reach out to us with any questions or concerns you may have with data collection and data maturity. We’re eager to help and our team has the experience and know-how to augment your data efforts.